In our recent webinar, Nuno Henrique Franco, PhD offers his unique perspective on improving reproducibility in biomedical research, emphasizing ethical laboratory animal care and refining scientific experiment design. What’s more, he provides insights into what causes these issues and offers solutions to enhance the future success of research. But before we dive in, there are some necessary terms to review.
Reproducibility: the ability of a researcher to duplicate the results of a prior study using the same materials used by the original investigator.1
Replicability: obtaining consistent results across studies answering the same scientific question.1
Translatability: translating the results we find in animal models to clinical practice in humans.2
To fully understand and improve the reproducibility crisis, which affects all scientific fields, it’s critical to understand these terms, the causes of irreproducible results, the consequences, and how to improve them.
What Are the Consequences of Poor Reproducibility in Biomedical Research?
The consequences of poor reproducibility in biomedical research are detrimental to people and animals everywhere. Franco makes this point starkly by sharing a study from Freedman (2015) investigating how reproducible preclinical data currently is. It shows that 50% of preclinical studies in the U.S. are irreproducible and that at least $28 billion goes to waste annually.
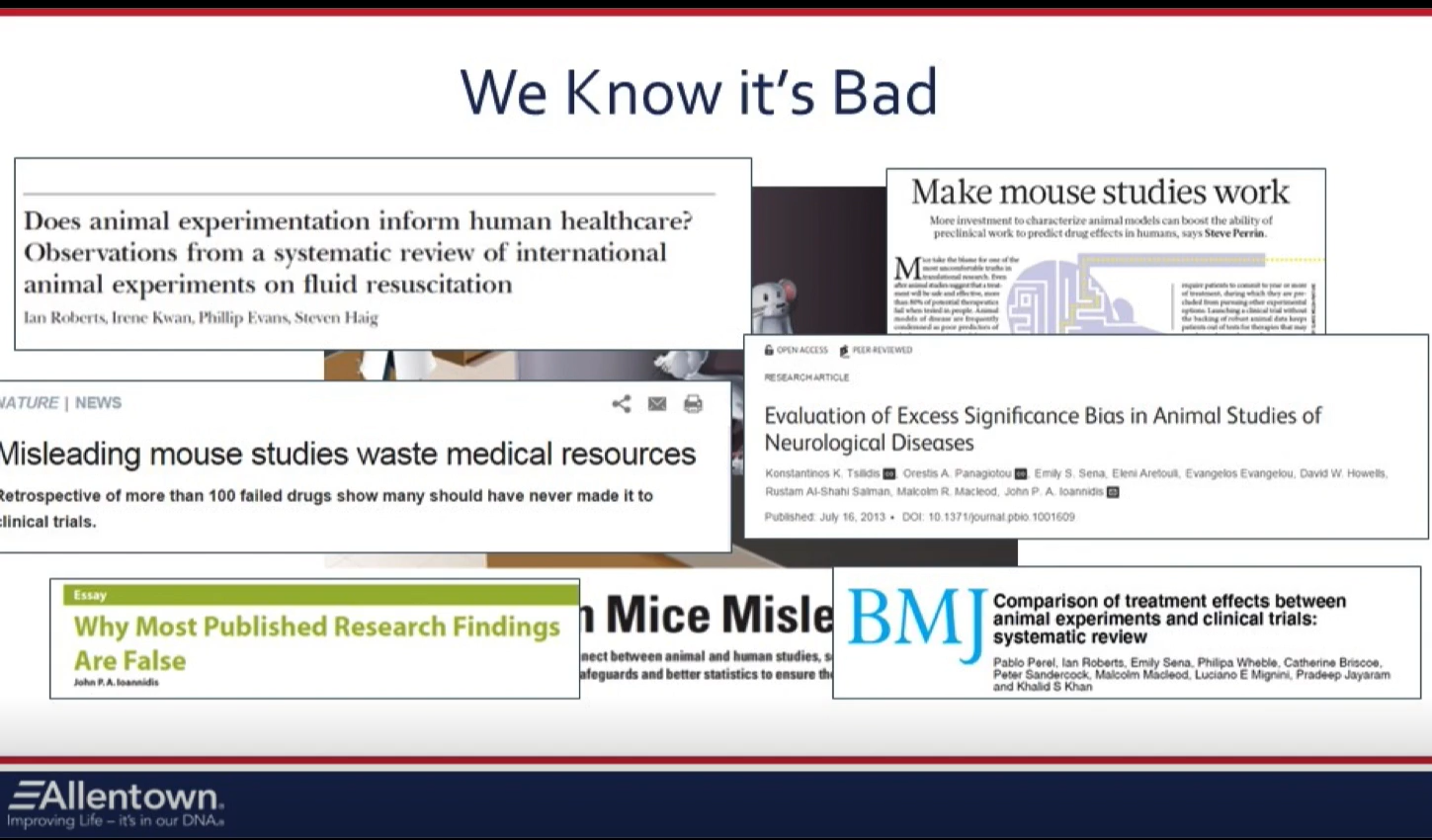
Franco doesn’t bring up this point to discredit or discourage researchers, but he mentions it to accentuate how imperative it is for the scientific community to do better. Billions of human and animal lives depend on reproducible research to translate into viable treatments, cures, and solutions. Poor reproducibility in research contributes to mistrust of science among the public and fewer cures. The consequence of poor reproducibility in biomedical research is the difference between life and death for many.
What Causes Insufficient Reproducibility in Biomedical Research?
Franco gives insights into what renowned Psychologist, Dorothy Bishop, describes as the four major threats to reproducible research. They are as follows:
- Publication bias
- Low statistical power
- P-value hacking
- HARKing (hypothesizing after results are known)
To the above, he added
- Poor research quality control for errors and biases in experimental design
- Flawed reward system in science
1. Publication Bias
According to Franco, one threat against reproducible science is publication bias. So, what is it? Publication bias is the failure to publish the results of a study based on the direction or strength of the study findings.3 For example, imagine a researcher hypothesizes that a particular drug will be effective in some way. Then, the researcher conducts her study and concludes that the drug was ineffective, contrary to her prediction. Failure to publish her work (because it was contrary to her initial hypothesis) – either because the researcher or the journal feels it doesn’t merit publication – is an example of publication bias.
Publication bias is problematic because it withholds valuable information. Furthermore, according to Franco, there is no such thing as a “negative” result. Even if a research result proves your hypothesis wrong, it is still valuable information. So, although it might be challenging to admit you’re wrong, or to publish something like “drug X does not work,” it’s crucial to put it on the record.
Franco calls for a culture shift that encourages the publication of “negative” research results. He explains some might have a drastic impact, and some might have a minor effect, but at least it will be on the record for future research, including meta-analyses synthesizing the available evidence.
2. Statistical Power
Power is the probability an experiment will detect a treatment effect. Power is low when the sample size is insufficient to detect an effect of a given size. According to Franco, not only do low-powered experiments have a higher likelihood of resulting in false negatives, but they also are more likely to report exacerbated estimates of a result, as one would expect when an effect is claimed to have been found even though the probability of finding it would be low.
According to Franco, false results lead to waste; it wastes animals, time, and money. Also, it lowers the predictive value of animal research in general. Additionally, it could even lead to wrongful closures of research facilities. That’s why it’s critical to ensure adequate sample sizes in research with animals. Statistical power is essential for more reproducible research.
3. P-Hacking Threatens Reproducibility in Biomedical Research
So, what is P-hacking? P-hacking is reanalyzing a dataset in multiple ways or even with additional replicas until you get the desired result. Franco admits that many researchers, including his past young self, are guilty of this sometimes. But at the end of the day, you’re tricking yourself. And, as Franco says, “it must stop if we are to achieve more reproducible research results.”
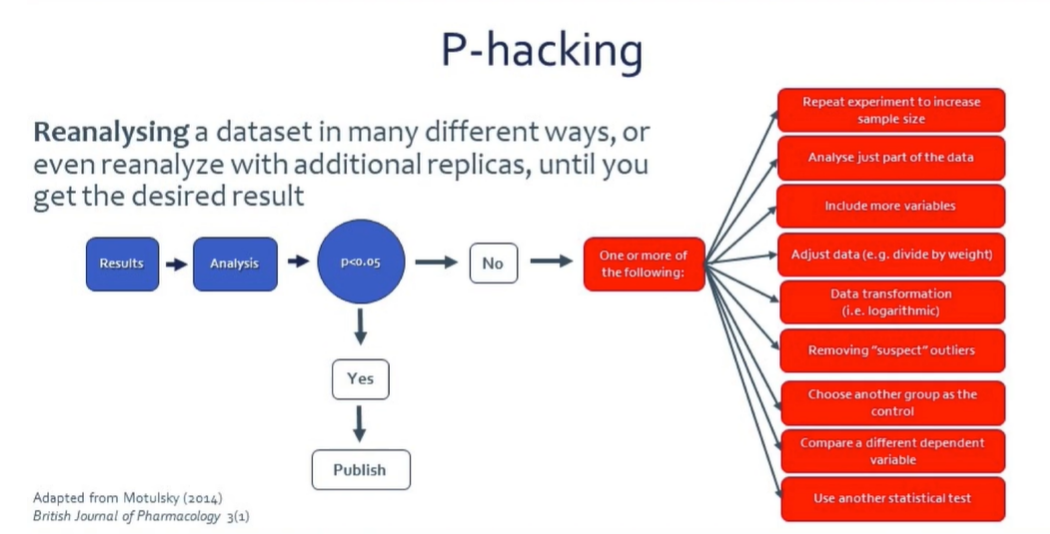
P-Hacking occurs when researchers – most often from not knowing any better – reanalyze or remeasure data upon not finding the expected results, “repairing” the experiment to increase the size, analyzing just one part of the data, adding more variables, adjusting data (e.g. to the weight of the animals), removing suspect outliers, comparing different dependent variables, and more. Treating data is often necessary for analysis, but this should be decided beforehand. Doing so upon not finding p<0.05 is never OK. It’s unethical and contributes to the reproducibility crisis.
4. HARKing Hurts Reproducibility in Biomedical Research
Franco compares HARKing to moving the goalposts in the middle of a soccer game. Essentially, it’s cheating! HARKing stands for hypothesizing after the results are known. Franco explains that if your data is inconsistent with your hypothesis, you still have a valid result! It is how science works, your results help you know whether you are right, or you are wrong, and you learn in both cases. Plus, it’s OK to state or offer possible explanations to test afterward! However, claiming that your new hypothesis was your original one without referring to it is never acceptable.
5. Poor research quality control of errors and biases in experimental design
According to Franco, there are many possibilities for common unaccounted errors and biases that go uncontrolled when planning and executing an experiment. The most prevalent four are as follows:
- Selection bias
- Performance bias
- Detection bias
- Attrition bias
But it doesn’t stop there. Here are more possible unaccounted errors and biases that could impact experimental design, such as:
- Sex bias
- Poorly designed controls
- Comorbidities
- Stress and distress
- Reporting bias
- Undisclosed conflicts of interest
According to Franco, these unaccounted-for biases in experimental design affect the internal validity of the research. Failing to control these biases has many unwanted adverse effects. And if it affects the internal validity of animal experiments, it leads to unreproducible results, thus affecting external validity, and misinforming experimental treatments’ value, in turn leading to low translatability of animal studies.
How to Improve Reproducibility in Biomedical Research
So, how can you combat Harking, P-hacking, and unaccounted-for biases? Franco explains there must be more training and education for researchers to improve reproducibility in animal research. For example, more education and training on statistics and experimental design could help improve things. Also, attending or giving more talks or lectures about reproducibility in research can help.
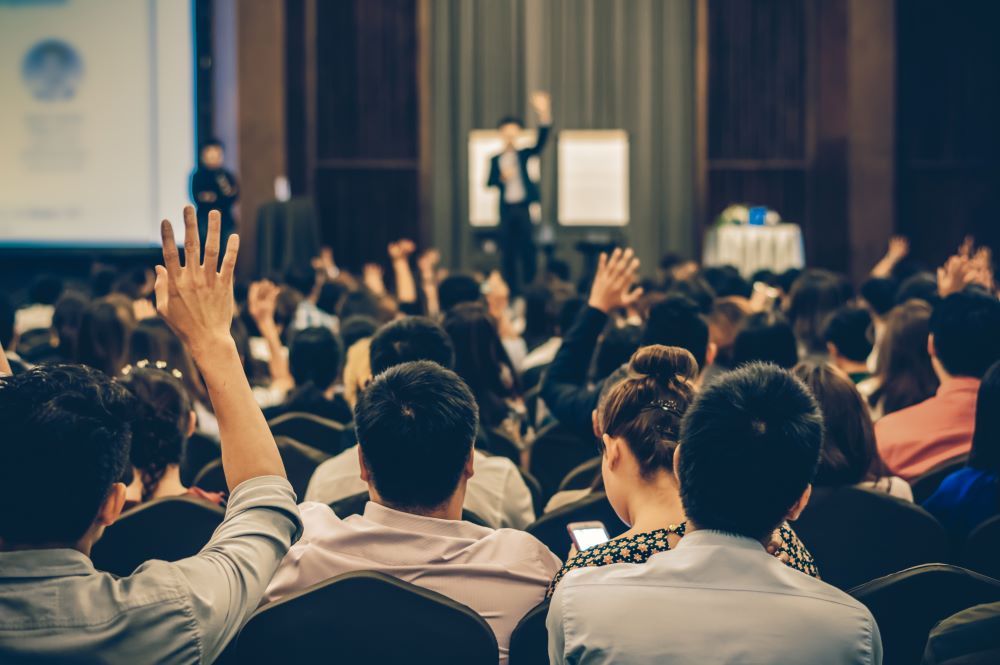
But ultimately, Franco believes researchers need to do a better job of planning, pre-registering, and reporting! Below are some additional tools that Franco recommends to help researchers accomplish just that.
1. PREPARE Guidelines
One of the tools Franco suggests using to help improve your research replicability is the PREPARE (planning research and experimental procedures on animals recommendations for excellence) Guidelines authored by NORECOPA, RSPCA, and UFAW.
PREPARE guidelines are inspired by the precise checklists used by pilots. These are the same checklists that ensure every plane takes off and lands with minimal issues. It focuses on precision, replicability, health, safety, and translatability. It helps encourage teamwork, communication, and coordination.
2. Open Science for Improved Reproducibility in Biomedical Research
Franco and many other leading experts believe that more transparency and openness in science is the best way to improve reproducibility in research. He urges that all aspects of science should be open, including data, protocols, and software. Everything should be completely transparent. He explains that honesty and transparency create more opportunities for growth and positive change.
More From Our Webinar: The Role of Lab Animal Caretakers
Caretakers not only play a central role in providing animals with care and improving animal welfare but also have a vital role in improving the reproducibility of animal studies! As Franco kindly points out, technicians and caretakers are the eyes and ears of research. For example, if something is off, including conditions, equipment, or care, the researchers rely on them to raise their hands and say something. It could make or break a study.
To learn more about the role of lab animal technicians and how they make a difference, read our recent blog story, “Lab Animal Technicians: The Heart of Biomedical Research.”
References:
- “Subject and Course Guides: Research Reproducibility: About.” About – Research Reproducibility – Subject and Course Guides at University of Illinois at Chicago, researchguides.uic.edu/reproducibility#:~:text=Reproducibility%3A%20%E2%80%9Cthe%20ability%20of%20a,used%20by%20the%20original%20investigator. Accessed 12 May 2023.
- Sciences, Engineering National Academies Of, et al. Reproducibility and Replicability in Science. National Academies Press, 2020.
- Nair, AbhijitS. “Publication Bias – Importance of Studies with Negative Results!” Indian Journal of Anaesthesia, vol. 63, no. 6, 2019, p. 505, https://doi.org/10.4103/ija.ija_142_19.